Historical Background
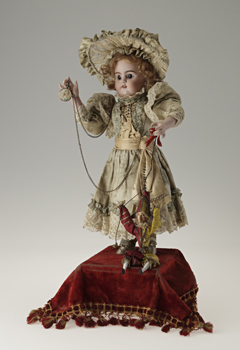
Long before the first computers were built, the idea of machines capable of acting like human beings fascinated thinkers around the world. These mythical creatures included the Greek figure Talos, a gigantic animated bronze warrior assigned to guard the island of Crete. A Chinese legend tells of a mechanical man, built by the engineer Yan Shi, that was presented to the king of the Zhou Dynasty in the 10th century BCE. At the heart of the Jewish tradition of the Golem is a creature made of clay who could carry out the commands of its creator through the aid of magic spells. More broadly, animism, or the belief that objects and non-human beings could possess an animating spirit allowing them to engage with the minds of humans, has been a strong component of many world religions, including North American Indigenous spiritual practices. These cultural contexts have underpinned the human fascination with creating artificial intelligence.
With access to precision metalworking technologies, many societies around the world began to develop automata, or mechanical devices able to partially mimic human behaviour. In 807, Harun al-Rashid, the Abbasid caliph in Baghdad, sent a spectacular mechanical clock complete with 12 mechanical horsemen as a gift to the Holy Roman Emperor Charlemagne. In The Book of Knowledge of Ingenious Mechanical Devices (c. 1206 CE), the Turkish mechanical engineer al-Jazari laid out plans for a mechanical orchestra powered by water pressure.
The European Renaissance saw Italian inventor and humanist Leonardo da Vinci (1452–1519) design a fully armoured mechanical knight, and mechanical figures were installed as part of church clocks in German cities such as Nuremberg. By the 18th century, French and Swiss craftsmen were producing automata that can still be seen in museums today. Famous examples of these include a doll-size mechanical boy able to write in ink, created by Swiss clockmaker Pierre Jaquet-Droz, and Frenchman Jacques de Vaucanson’s “digesting duck,” a mechanical bird that impressed audiences by ingesting real food — and seemingly expelling it afterward. French and English colonists brought automata to Canada: for instance, a mechanical doll from 19th-century French craftsman Léopold Lambert is part of the collection of Montreal’s McCord Museum.
First Uses of Electronic Computing in AI Research
While mechanical automata could repeat particular human behaviours, their “intelligence” — their ability to accomplish complex, adaptive and socially contextual goals — proved simplistic and one-dimensional. However, with the development of electronic digital computers in the 1930s and ‘40s, many scientists became convinced that computing machines could be made to exhibit intelligent behaviour by replicating the functioning of the human brain.
Early computing pioneers, such as the United Kingdom’s Alan Turing, were fascinated by the ways digital machines could be understood as similar or different to human minds, and by how they might be programmed to think (and perhaps even feel) like humans. In 1943, American neuroscientist Warren S. McCulloch and logician Walter Pitts formulated a theoretical design for an “artificial neuron,” marking one of the first attempts to mimic the workings of the brain using electric charge.
As soon as electronic computers became more widely accessible to academic, government and military researchers at the end of the Second World War, computing researchers began to program them to do things that had been considered reserved for the human mind. These tasks included solving complex mathematical problems and understanding sentences in languages like English, French or Russian.
Did you know?
Chess was among the tasks that most interested AI researchers, who began to write chess-playing programs in the 1940s. The idea of chess-playing automata had been introduced in the 18th century, when a “Mechanical Turk” toured the royal courts of Europe, impressing onlookers with its apparent ability to play chess. The Mechanical Turk was later revealed to be a hoax (a human operator was squeezed inside its mechanism), but chess remained an obvious logical problem for AI to tackle. The defeat of world chess champion Gary Kasparov by IBM’s Deep Blue computer in 1997 was a landmark for AI research.
In 1950, Turing developed what he called the “imitation game,” which is now better known as the “Turing Test.” Turing first proposed a scenario where a human interrogator sitting in one room had to guess whether two other individuals sitting in another room were a man or a woman solely based on their written responses; Turing then suggested the man be switched with a computer. “Will the interrogator decide wrongly as often when the game is played like this as he does when the game is played between a man and a woman?” Turing asked. If so, he declared, a machine could be understood to think.
The Turing Test has become simplified to refer to the ability of a computer to fool a human into thinking it is also human a high percentage of the time. However, Turing’s original formula points to the ways human gender and other forms of identity can challenge simplistic or non-diverse understandings of human intelligence.
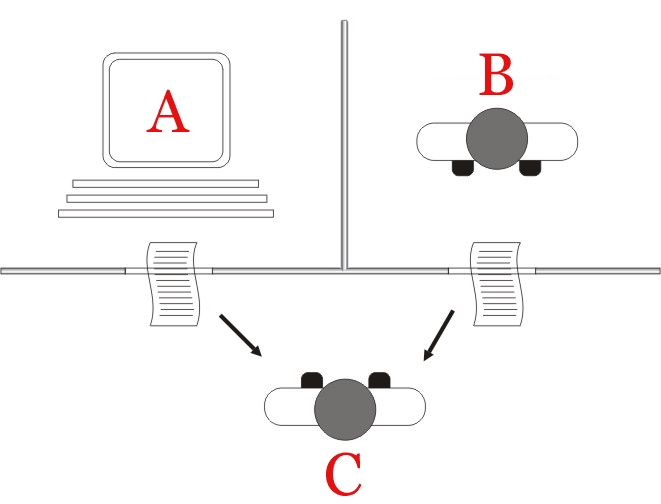
Birth of the AI Field
Artificial intelligence took shape as a subfield of computer science, cognitive science, and engineering in the early 1950s. One important moment in its development took place at New Hampshire’s Dartmouth College in the summer of 1956. A number of academics working at US institutions — including Harvard’s Marvin Minsky, the Carnegie Institute of Technology’s Allen Newell and Herbert Simon, Claude Shannon of Bell Labs and Dartmouth’s own John McCarthy — gathered there for two months to “find how to make machines use language, form abstractions and concepts, solve kinds of problems now reserved for humans, and improve themselves.” According to McCarthy, early AI research focused on how to “consider the computer as a tool for solving certain classes of problems.” AI was therefore “created as a branch of computer science and not as a branch of psychology,” a decision that profoundly shaped the history of the field by formally separating it from the social sciences.
Whether and how to compare artificial intelligence to human intelligence continues to be a focus of intense scholarly debate. This debate also underpins an important conceptual division in AI research: between the search for artificial general intelligence and applied artificial intelligence. Artificial general intelligence, or “strong AI,” refers to machine systems with cognitive abilities at or beyond those of a human. Applied artificial intelligence, or “weak AI,” refers to machine systems able to perform particular tasks inspired by human cognition.
Researchers in the 1950s and ‘60s began to study machine translation, machine learning and symbolic logic approaches to AI. Early work in AI tended to cluster around two approaches. One was to develop general-purpose symbolic programming languages, including ones that could understand commands in human languages like English. The second was to encode large amounts of specialized knowledge and use the machine to draw relevant inferences from this knowledge. The technical limitations of the computers of the time challenged early researchers. Exhaustive search and statistical learning methods could not complete cognitive tasks at speeds anywhere near what a typical human could do.
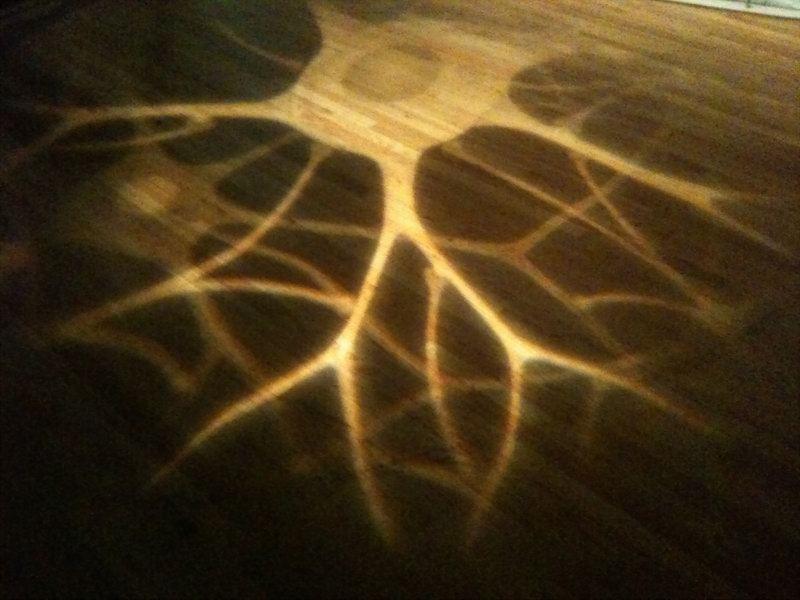
From Golden Age to “AI Winter”
Despite lofty ambitions and some impressive initial successes, the major practical result of early AI research was the development of basic computing tools. Some of these tools, which we often take for granted today, include list-processing programming languages like LISP (invented by McCarthy from 1956 to 1958 and still in use) and time sharing (enabling multiple individuals to make use of one computer’s processing capabilities at the same time).
Because of our bilingual heritage, Canadians have a long tradition of research in machine translation. In the mid-1970s, a group at the Université de Montréal developed what was at that time the only fully automatic, high-quality translation system in continuous daily use. Known as the MÉTÉO system, it translated the formulaic weather forecasts at the Canadian Meteorological Centre in the Montreal suburb of Dorval. A far more sophisticated system was later developed by the same group for translating aircraft maintenance manuals. Even though the quality of translation was considered high, government funding for the latter project was abandoned in 1981 because the cost of editing the system’s translations and updating its dictionary made it uneconomical to use. The MÉTÉO system, however, was used until 2001.
In 1973, the Canadian Society for Computational Studies of Intelligence (now the Canadian Artificial Intelligence Association) was founded at the University of Western Ontario (now Western University) by academic researchers from across the country.
The 1970s also brought the first commercial use of machines that could reason from a base of knowledge provided by human experts. Such “expert systems” supported certain narrow areas of expertise, including specialized medical diagnosis, chemical analysis, circuit design and mineral prospecting. Canadian mining expert Alan Campbell collaborated on a well-known early AI prospecting system, PROSPECTOR, developed at Stanford University in California.
Other AI programs emerged in the 1970s that could “understand” a limited range of spoken or typed language. Some could also visually examine layouts, such as metal castings or integrated circuit chips on an assembly line.
Yet the mixed success of automated machine translation during this period was disappointing to government funding agencies. The high cost and narrow feasibility of actual AI applications had fallen far short of many researchers’ optimistic claims. Beginning in the mid-1970s, the first “AI Winter” set in, as governments in North America and Europe scaled back their funding for research into machine intelligence.
The 1980s and “Fifth-Generation” Computing
In the early 1980s, Japan, followed closely by the United Kingdom and the European Economic Community, announced major national programs to develop what were sometimes called “fifth-generation” AI computer systems. They were billed by the Japanese government as an important step in technological sophistication beyond the “fourth-generation” microprocessor computers developed in the late 1970s. These AI systems were grounded in the model of encoding specialized knowledge in machines. They were also based on parallel processing: the simultaneous use of multiple processors to analyze data on a large scale. Such improvements in computer hardware and software led researchers around the world to predict significant advances in AI.
Amid the enthusiasm for fifth-generation computing, a number of private groups and government departments began to recognize the importance of AI research to Canada’s future. They also recognized the need to support academic and industry research on AI in Canada. The Canadian Institute for Advanced Research (CIFAR) was founded in 1982 to promote groundbreaking research in strategically important areas. It selected AI and robotics as its first focus with the Artificial Intelligence, Robotics, and Society program, launched in 1983. Research groups specializing in AI were also already active in several provinces (notably Alberta, British Columbia, Ontario and Quebec).
In the late 1980s, a group of several dozen Canadian companies formed Precarn Associates, a consortium promoting long-term applied research into AI technologies. Facilitating collaboration between industry, government and university researchers, it worked to develop new commercial applications of AI and helped Canadian companies compete in global markets. Precarn included not only small high-technology industries but also large steel and mining companies and public utilities. It was renamed Precarn Incorporated in 2001. During the mid-2000s, it faced funding constraints under Prime Minister Stephen Harper’s Conservative government, which ultimately led to its dissolution. Precarn ceased operations in 2011.
By the late 1980s, desktop computers utilizing fourth-generation microprocessor chips from firms like Intel had become more computationally powerful and had found a wider range of uses in everyday life. This caused a collapse in the business market for specialized fifth-generation computers designed with AI systems in mind. The ensuing second “AI Winter” saw government funding for artificial intelligence research cut again.
1990s and 2000s: The Rise of Machine Learning and Deep Learning
Artificial intelligence research continued in academic institutions despite the funding cuts of the late 1980s. The 1990s saw increased research activity in the subfield of AI research known as machine learning (ML). Machine learning uses statistical analyses of large amounts of data to train digital systems to execute particular tasks. Examples of such tasks include retrieving all digital images of a cat within a set, or successfully identifying and interpreting words in a particular human language (known as Natural Language Processing, or NLP). With the increasing amount of digital data available as the Internet expanded into people’s everyday lives, ML soon became a leading subset of AI research.
Machine translation was one subfield of ML/NLP that continued to benefit from Canadian expertise. In 1992, a major machine translation conference in Montreal saw a spirited debate between two strands of machine translation research: “rationalists,” who used linguistic theories to structure computer translation, and “empiricists,” who used large-scale statistical processing of enormous data sets. Research at IBM, which typified the empirical approach, had used Canada’s bilingual parliamentary record, the Hansard, as the material for its translation work.
Canadian universities were early to invest in ML research, in part because of CIFAR’s ongoing support for the field. In 1987, the University of Toronto hired Geoffrey E. Hinton, a pioneer in the ML subfield of deep learning (the use of artificial neural networks to analyze data). In 1993, the Université de Montréal hired ML researcher Yoshua Bengio, who had received his PhD from McGill University two years earlier. And in 2003, the University of Alberta hired American Rich Sutton, a pioneer in the ML subfield of reinforcement learning.
Did you know?
Geoffrey E. Hinton shared the 2024 Nobel Prize in Physics with John Hopfield of Princeton University. According to the Royal Swedish Academy of Sciences, Hinton and Hopfield were awarded the prize “for foundational discoveries and inventions that enable machine learning with artificial neural networks.” (See also Nobel Prizes and Canada.)
In 2004, CIFAR helped catalyze scholarly work in deep learning with its Neural Computation and Adaptive Perception program, under Hinton’s direction. The program (now known as Learning in Machines and Brains) sought to revive interest in neural networks by bringing together experts from computer science, biology, neuroscience and psychology. Along with many colleagues and collaborators, Sutton, Bengio and Hinton were central to creating the conditions for Canada’s increasing importance as a centre for AI research.
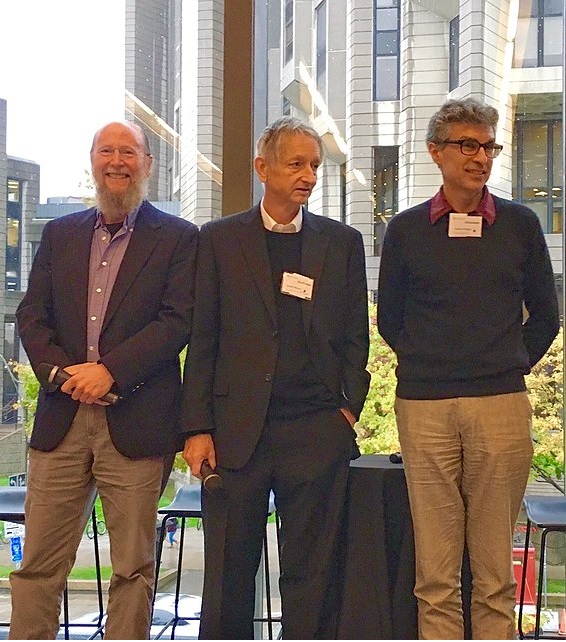
Did you know?
Yoshua Bengio, Geoffrey Hinton and fellow computer scientist Yann LeCun won the 2018 A.M. Turing Award for their groundbreaking research on deep neural networks. Given out by the Association for Computing Machinery (ACM), the $1 million award has been called the “Nobel Prize of computing.” The ACM cited the three men’s work as the foundation of rapid leaps in technologies such as computer vision, speech recognition and natural language processing. In interviews following the award, Bengio and Hinton warned about the use of AI in weapons systems and called for an international agreement to limit this type of warfare.
2005 to Present: Canada Takes a Global Lead
Since 2005, there has been a global resurgence in commercial and academic interest in AI, now often synonymous with machine learning techniques. Canada is at the forefront of this new enthusiasm for AI, with leading academic research clusters at Canadian institutions including the University of British Columbia, University of Alberta, University of Waterloo, University of Toronto, McGill University and Université de Montréal. Canada’s embrace of diverse intellectual talent from around the world has also been a major factor in the country’s success at attracting international investment in AI technologies.
Canada has sought to capitalize on its existing strengths in AI research. In its 2017 federal budget, the Liberal government of Prime Minister Justin Trudeau announced $125 million for a five-year Pan-Canadian Artificial Intelligence Strategy coordinated by CIFAR. The strategy funded the creation of three new AI institutes: the Alberta Machine Intelligence Institute (AMII) in Edmonton, the Vector Institute in Toronto and the Montreal Institute for Learning Algorithms (MILA). It also aims to support a national research community focused on AI, increase the number of AI researchers in Canada, and “develop global thought leadership on the economic, ethical, policy and legal implications of advances in artificial intelligence.” Ahead of the 2018 G7 Summit in Charlevoix, Quebec, Trudeau and French president Emmanuel Macron committed to create an international study group on inclusive and ethical AI. Canada also hosted a G7 conference in December 2018 focused on AI’s responsible adoption and businesses applications.
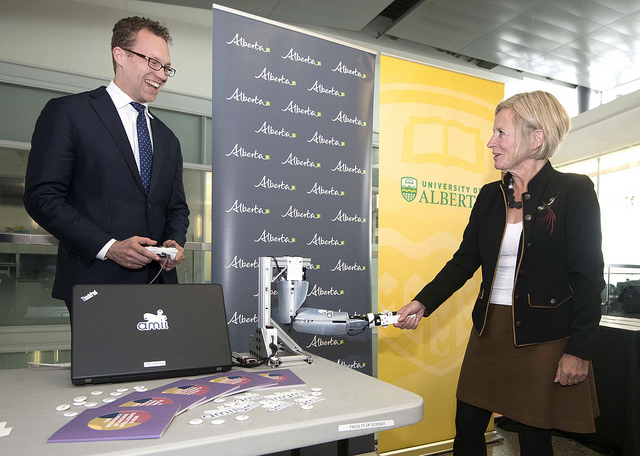
Canadian AI Companies
Canadian companies are working to translate this expertise and enthusiasm around AI technologies into robust business. One such success story is Maluuba, a company founded by graduates of the University of Waterloo in 2011. Maluuba develops AI systems capable of understanding and interpreting natural human language. With a deep learning research team focused on natural language understanding, the Montreal-based company was purchased by Microsoft in 2017 and transformed into a Microsoft Research lab (see also Microsoft Canada Inc).
Another is Borealis AI, a research centre founded by the Royal Bank of Canada in 2016. Borealis AI applies machine learning technology to financial services. Its areas of focus include reinforcement learning, natural language processing and ethical AI. It has offices in Toronto, Montreal, Vancouver and Waterloo.
Canadian AI start-ups in Montreal and Toronto develop applications ranging from conversational marketing to meal delivery and online retail. In January 2018, Canadian mobile communications company BlackBerry signed an agreement with Chinese search-engine provider Baidu to jointly develop machine learning technology for self-driving cars. Other global digital firms like Facebook, Google, Samsung and NVIDIA have also opened research centres in Montreal and Toronto. DeepMind, an arm of Google, has research labs in Edmonton and Montreal.
Opportunities and Challenges of AI
Artificial intelligence is already impacting the everyday lives of Canadians as AI technologies become increasingly integrated into the digital systems used by governments and businesses alike. Much of the public conversation about (and marketing of) AI references the visions of “strong AI” in myths, legends and science fiction. But applied AI technologies like machine learning and intelligent agents (e.g., chatbots on customer service web pages or virtual agents like Apple’s Siri on smartphones) are already part of Canadian society. These technologies have a variety of potential positive uses in commercial and government contexts: a 2018 report by the Treasury Board of Canada Secretariat notes the potential for AI technologies to assist Canadians and businesses with routine transactions via virtual service agents, to monitor industries for early warning signs of regulatory non-compliance, and to shape public policy by facilitating new insights into government data.
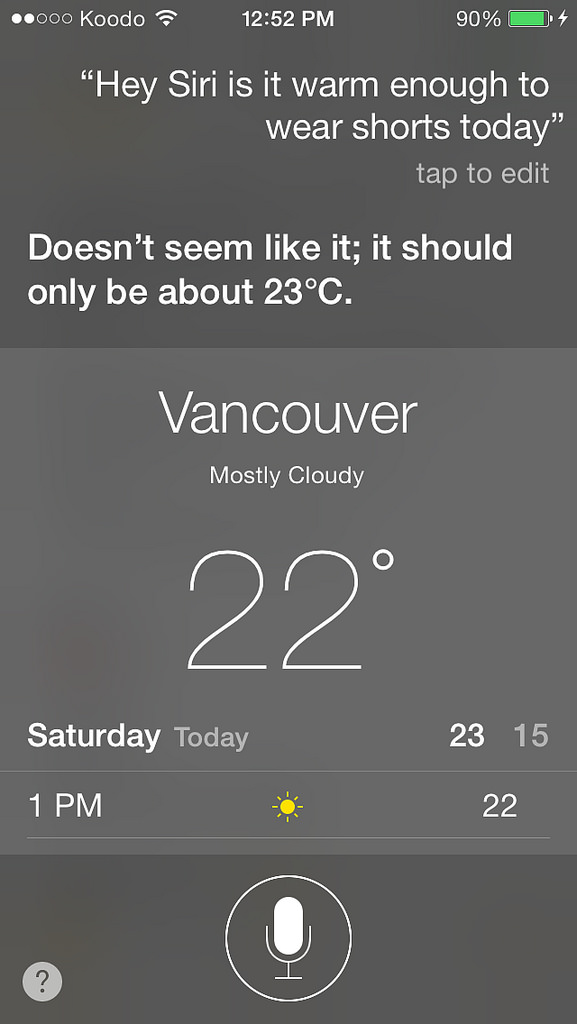
Yet AI technologies also pose a number of looming challenges to Canadian society. One major concern is the potential for job losses created by a new wave of “white-collar” automation. Another is the potential for machine-learning systems in fields such as criminal justice to reinforce existing racial and other biases. Techniques such as machine learning are based on large amounts of digital data about the world, and it is difficult to avoid transferring and amplifying the racism, sexism and other human prejudices expressed in that data into AI systems.
By the same token, AI technologies reliant on large amounts of digital data raise considerable privacy concerns, potentially allowing large institutions to make assumptions about personal details Canadians don’t want shared. Assumptions about an individual’s health could raise their insurance rates, for example, or assumptions about their behaviour could be used against them in a court of law. Even well-designed machine-learning systems can create inequality or discrimination if they’re used in unfair or unjust contexts, as mechanisms to cement or exacerbate societal inequalities.
Did you know?
Some units of the Royal Canadian Mounted Police (RCMP) used a facial recognition software called Clearview AI to investigate crimes. Clearview AI was designed to find details about a person in a photo by scanning a database of billions of images collected from social media and other sites. Before Clearview AI’s client list was hacked and leaked to the media in early 2020, the RCMP had denied using facial recognition. Other police forces in Canada also admitted to having used the software following the leak. Amid concerns about privacy, the RCMP said it would only use the software in urgent cases, such as identifying victims of child sexual abuse. The RCMP stopped using Clearview AI once the company ceased operations in Canada in July 2020. A June 2021 report released by the Office of the Privacy Commissioner (OPC) found that the RCMP had violated the Privacy Act by using Clearview AI.
Canadian digital technology companies, including those working in AI, have considerable work to do in increasing the gender and racial diversity of their workforces. They must also do more to engage members of society who are technologically underserved. Many Canadians, including Indigenous people in many rural or remote communities, have limited access to digital technologies. (See also: Computers and Canadian Society; Information Society.)
Responsible Development of AI
As more and more companies and governments have deployed AI technologies, concerned members of civil society groups, professional associations, and even technology firms themselves have increasingly worried about the ethics of using these technologies or even developing them at all. In 2017, a group based at the Université de Montréal unveiled the first draft of the Montreal Declaration for a Responsible Development of Artificial Intelligence, which outlines broad principles for developing AI in the interests of humanity. The document declares that AI should ultimately promote the well-being of all sentient creatures, informed participation in public life and democratic debate.
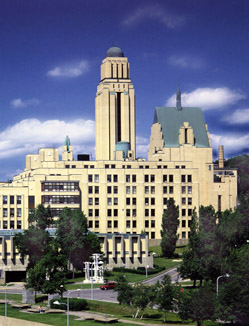
Canadians face challenging but potentially fruitful national conversations about the roles of AI technologies in different sectors and the ways they could be used to strengthen Canada’s social fabric. One focus of CIFAR’s Pan-Canadian Artificial Intelligence Strategy is to study the effects of AI on Canadian society. Sociologists, anthropologists, lawyers, economists and historians all have roles to play alongside computer scientists and engineers in understanding these implications. So, too, do Canadians influenced by these technologies in their everyday lives.